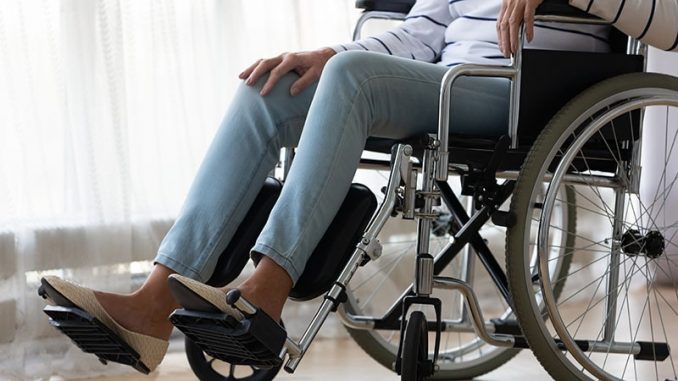
BOSTON – In patients with primary progressive multiple sclerosis (PPMS), machine learning can predict progression with reasonable accuracy on the basis of the blood transcriptome, according to a proof-of-concept study presented at the 2023 annual meeting of the American Academy of Neurology.
The accuracy was sufficient for the lead author of the study, Michael Gurevich, PhD, head of the Neuroimmunological Laboratory in the Multiple Sclerosis Center of Sheba Medical Center in Ramat Gan, Israel, to say that it is already clinically viable even as the tool is still evolving.
“We are looking at larger sample sizes to improve the accuracy and generalizability of our model, but we can use it now to inform treatment decisions,” Dr. Gurevich said.
In patients with PPMS who have a highly variable course, the model predicts disability progression with an accuracy of approximately 70%, according to the data he presented. He said he believes this is already at a level that it is meaningful for a risk-to-benefit calculation when considering treatment.
Machine learning analyzes blood samples
The study pursues the concept that the genetic information governing highly complex pathophysiological processes is contained in RNA sequencing. While multimodal omics generate data that are too complex for human pattern recognition, there is a growing body of evidence, including that provided by this study, to suggest that machine learning can employ these same RNA profiles and predict disease activity.
In this study, blood samples were collected from patients who participated in the phase 3 clinical ORATORIO trial that led to approval of ocrelizumab for PPMS. Analyses were conducted only on blood samples from those randomized to placebo, who, like those in the active treatment arm, were evaluated at baseline and at 12-week intervals for more than 2 years.
After development of a prediction model and creation of a training dataset, machine learning was applied to the deep sequencing of the blood transcriptome data for predicting two endpoints. One was disease progression at 120 weeks defined as a 1 point or more increase in the Expanded Disability Status Scale (EDSS) among patients with confirmed disability progression for at least 12 weeks (12W-CDP).
The other was change at 120 weeks in brain morphology defined as a 1% or more reduction in brain volume (120W PBVC).
The peripheral blood samples were subjected to RNA sequencing analysis (RNA-Seq) using commercially available analysis techniques. The prediction model for the disability endpoint was based on data generated from the blood transcriptome of 135 patients of which 53 (39%) met the endpoint at 120 weeks. The prediction model for the change in brain morphology was based on the blood transcriptome from 94 patients of which 63 (67%) met the endpoint.
On the basis of 10 genes that most significantly differentiated those who met the disability endpoint from those who did not, machine recognition of patterns after training was able to predict correctly the outcome in 70.9%. The sensitivity was 55.6%, and the specificity was 79.0%. The positive and negative predictive values were 59.0% and 76.8%, respectively.
On the basis of the 12 genes the most significantly differentiated those that reached the 120W PBVC endpoint from those who did not, machine learning resulted in a correct prediction of outcomes in 75.1%. The sensitivity was 78.1%, and the specificity was 66.7%. The positive and negative predictive values were 83.3% and 58.8%, respectively
Typical of a PPMS trial population, the mean age of the patients was about 44 years. The mean disease duration was about 6 years. The majority of patients had an EDSS score below 5.5 at baseline. The baseline T2 lesion number was approximately 50.
If further validated by others and in larger studies, this type of information could play a valuable role in PPMS management, according to Dr. Gurevich. Now that there is an approved therapy for PPMS, it can help clinicians and patients determine whether to initiate treatment early to address the high risk of progression or delay treatment that might not be needed.
A useful tool
In the field of MS, most of the studies performed with machine learning have focused on the analysis of radiological images. However, others are now looking at the blood transcriptome as a potential path to better classifying a highly complex disease with substantial heterogeneity in presentation, progression, and outcome.
For example, machine learning of the blood transcriptome has also shown high accuracy in the diagnosis and classification of MS in patients with clinically isolated syndrome (CIS). One study, published in Cell Reports Medicine, was led by Cinthia Farina, PhD, Institute of Experimental Neurology, IRCCS San Raffaele Scientific Institute, Milan.
Although she did not hear the presentation by Dr. Gurevich, Dr. Farina is enthusiastic about the potential for machine learning to help manage MS through the analysis of the blood transcriptome. “I do believe that transcriptomics in peripheral immune cells may become a useful tool for MS diagnosis and prognosis,” she said.
In her own study, in which machine learning algorithms were developed and trained on the basis of peripheral blood from patients with CIS, the tool proved accurate with a strong potential for being incorporated into routine clinical management.
“Machine learning applied to the blood transcriptomes was extremely efficient with a 95.6% accuracy in discriminating PPMS from RRMS [relapsing-remitting] MS,” she reported.
Dr. Gurevich has no potential financial conflicts of interest to report. He reported funding for the study was provided by Roche. Dr. Farina reports financial relationships with Merck-Serono, Novartis, and Teva.
This article originally appeared on MDedge.com, part of the Medscape Professional Network.
Source: Read Full Article